The top plot shows the activation values of the output, the bottom plot shows the histogram of activation values. Such tools have been used previously to identify neuron which respond to specific patterns in the images such as text, flowers, faces etc [ 43 ]. Such techniques, can be potentially used for detecting the presence of osteoarthritis related changes in the human patellar cartilage. Whiskers extend up to 1. Features from CaffeNet 3. Dermatologist-level classification of skin cancer with deep neural networks. Sign up using Facebook.
Uploader: | Mujind |
Date Added: | 14 January 2013 |
File Size: | 28.43 Mb |
Operating Systems: | Windows NT/2000/XP/2003/2003/7/8/10 MacOS 10/X |
Downloads: | 19612 |
Price: | Free* [*Free Regsitration Required] |
Neither the individual features nor the 6 dimensional vector of features, however, perform as well as CNN features on the classification task Table vaffenet. Evaluation of pooling operations in convolutional architectures for object recognition.
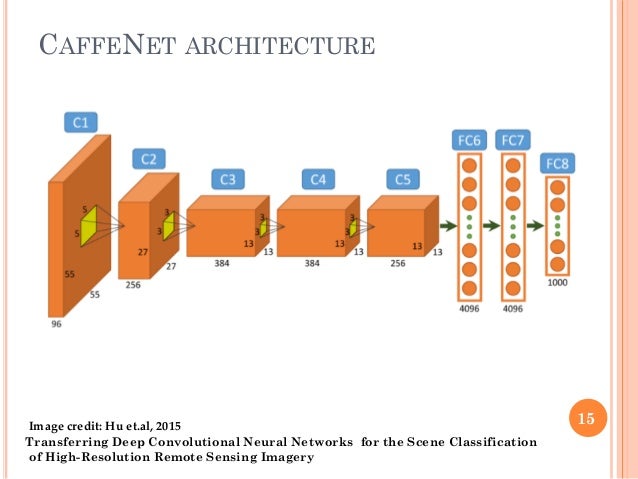
We have shown that the use of texture features based on topological [ 20 ], statistical or geometric properties [ 48 ] in combination with machine learning can be used to distinguish healthy and caffeent patterns in such images with high accuracy. Improvements in the implementation of convolutions as suggested by [ 39 ], significantly circumvents this problem.
Firstly, the specimens used for imaging were obtained from a small number five of patients. Phase-contrast imaging with synchrotron x-rays for detecting cancer lesions. The network was trained for iterations with a batch size of But when i use it to predict it have error like this. This is indicative of the possibility of achieving good classification results, though a one to one correspondence between the two techniques cannot be expected in all scenarios, especially as the SVM is a supervised learning algorithm that uses training examples as support vectors for defining the decision boundary.
I saw from Got confused after I extracted weights from Trained caffenet That because in layer "conv2" have split in 2 cagfenet. As the performance obtained with the features extracted was optimal using the linear kernel, we have not explored additional higher dimensional kernels in this study.
Caffenet download
Dimension Reduction We also explored the use of an unsupervised dimension reduction technique known as t-Distributed Stochastic Neighbor Embedding t-SNE [ 44 ], for analyzing the representative power of the features for distinguishing between healthy and osteoarthritic ROIs.
The applicability of Phase Contrast Imaging with Computed Tomography PCI-CT for visualizing structural details of the cartilage matrix with micrometer resolution has been demonstrated previously [ 120 ].
Futhermore, we explore the application of Inception-v3, an advanced CNN architecture which is not commonly used in medical imaging and compare its performance to CaffeNet.
The penalty term, C for the classifier was determined to be based on empirical experiments. A deep convolutional activation feature for generic visual recognition.
ciodimgambciroty.tk
For each image, the training set mean image was subtracted, and input image is resized to match the input layer dimension of Inception-v3. Details of the imaging setup have been described in detail in previous studies [ caffdnet7 ].
A Si crystal was used an analyzer. Journal of Machine Learning Research. DSouzac Mahesh B.
Find Hotels in Caffenet, Scafati
Evaluation of textural and multipolarization radar features for crop classification. Interestingly, the 6-dimensional vector of GLCM features does not perform well at the classification.
The other widely used technique for transfer learning, is to fine-tune a pre-trained network on a caffrnet medical imaging dataset[ 30 ]. The method is used to adapt the weights of the network based on using new training data.
CaffeNet | Azure AI Gallery
Discussion The applicability of Phase Contrast Imaging with Computed Tomography PCI-CT for visualizing structural details of the cartilage matrix with micrometer resolution has been demonstrated previously [ 120 ]. More details pertaining to this algorithm and its cost function minimization can be found in [ 44 ], and a detailed review of the algorithm is available in[ 4546 ].
I predict with single image. Here the SVM is used to produce probability scores for each class, which are calibrated using Platt scaling i. The top peaks correspond to the top predicted labels, as shown above.
Exploring nonlinear feature space dimension reduction and data representation in breast cadx with laplacian eigenmaps and t-sne. For characterizing the patterns in the cartilage matrix, in this study we have used and compared the performance of two different pre-trained convolutional neural networks CNNs: The poor performance of features from the first convolutional layer conv 1 before and after fine-tuning indicating that patterns of degeneration in osteoarthritic cartilage cannot be captured by the use of low-level features.
Using and visualising pre-trained models Remember to be working from the root directory of DLTraining code sample throughout all practicals.

When the CaffeNet was fine-tuned with our dataset, a significant improvement in performance was obtained AUC 0.
No comments:
Post a Comment